Understanding the process of interleaving between driving and non-driving task
In this project, we analyzed the process of interleaving between driving and non-driving tasks in a conditionally automated vehicle. We used a driving simulator to simulate manual driving and SAE level 5 automated driving and examined how drivers switch to non-driving task when the vehicle goes to automated driving. We analyzed the stages of interleaving, the order of the stages, time spent on each stage, driving performance and performance of non-driving task in different stages.
Speed Anomalies and Safe Departure Times from Uber Movement Data
We built a model to predict traffic speeds, which let us find anomalies on individual road segments. The Uber Movement datasets for 2018 and 2019 was used in the analysis. We found that speed anomalies mostly occur during rush hours and that longer than usual travel times are more frequent compared to shorter than usual travel times. We also found that speed patterns on some routes do not follow the conventional commute speed pattern. We used the speed statistics to compute safe departure times such that a traveler would likely reach their destination by a certain time with some pre-specified probability.
Driver transitions in automated vehicles
The focus of the research work is to understand the transition stages during the transfer of control in an automated vehicle. We designed an experiment with different take-over request times, Alerts, and non-driving tasks. From the experimental data, the driver’s order of a series of transition stages was found during take-over in an automated vehicle. Results showed that the drivers interleave longer between driving and non-driving task given higher take-over request times, and different take-over request time has an impact on driver transition stages. More analysis on understanding driving behavior and transition stages under various driving conditions to be examined.
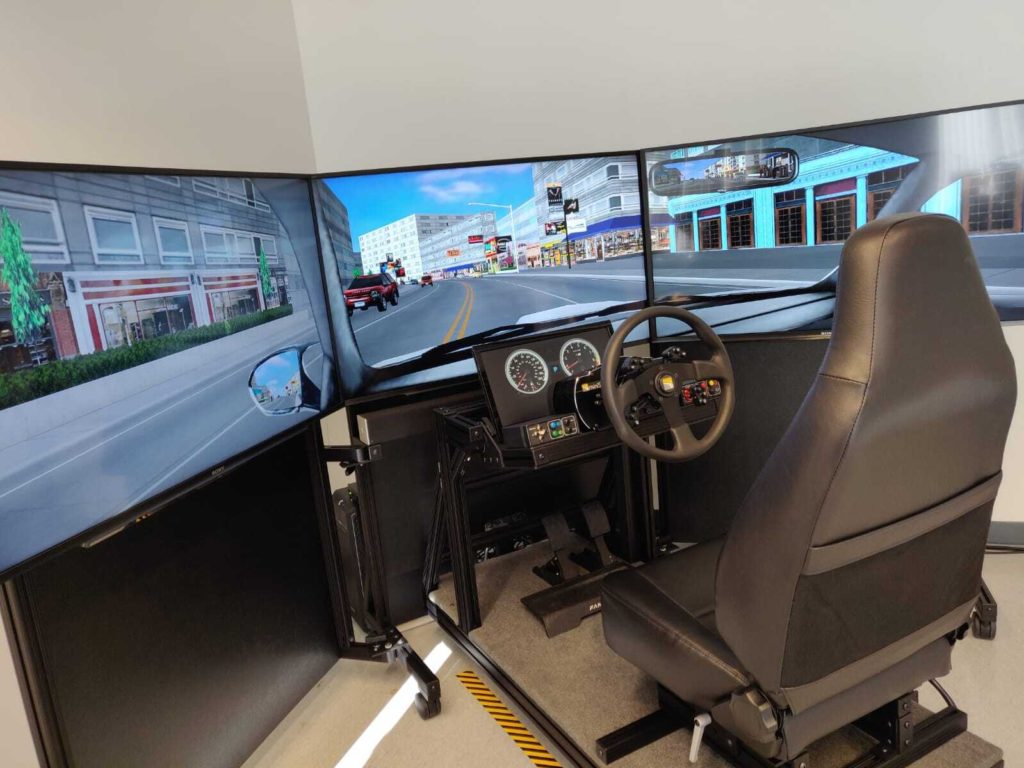
Computational identification of visual attention of interactive surfaces
This study involves identifying the gaze location of people interacting with a multi-touch display. We introduce a computational method for estimating visual attention. We implemented a method using head-worn eye-trackers to collect gaze data and used that data to create a neural network model to estimate the gaze location. We also investigated the design considerations for implementing a neural network model for this problem.